Project
Powering the Orkney Cloud
Modeling and Understanding Curtailment in Orkney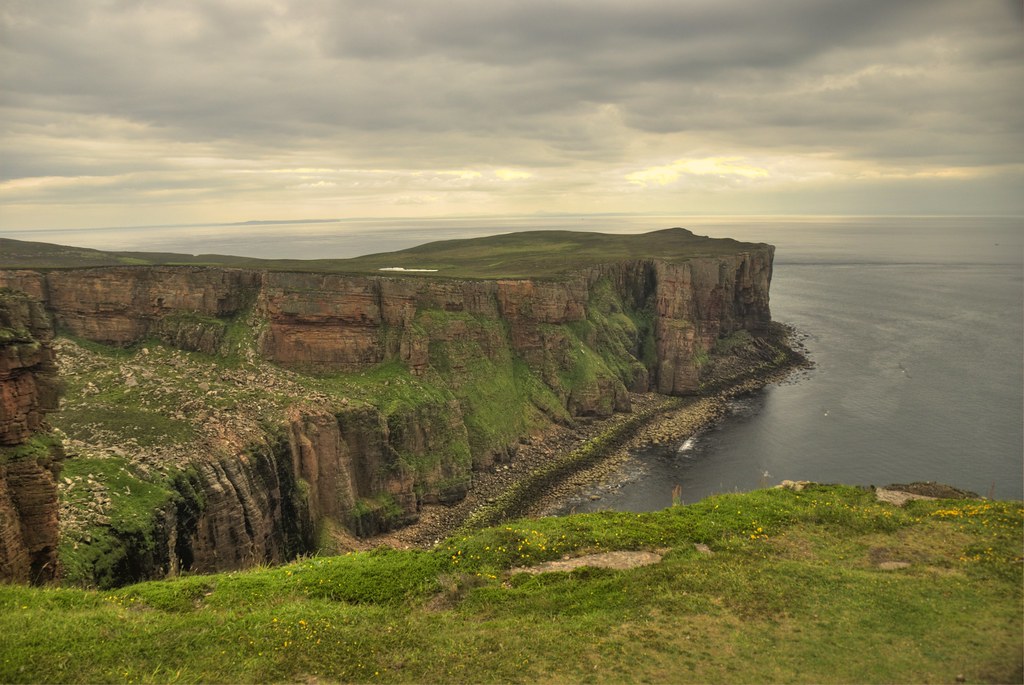
Abstract:
In this thesis we explore the idea of an Orkney Cloud data center, a decentralized data center running on the surplus renewable energy otherwise curtailed in the power grid of Orkney, Scotland. The concrete issue addressed is the unclarity of the logic governing the Active Network Management (ANM) curtailment system in Orkney. The focus is to gain the needed understanding of the ANM to design and implement systems that can adapt their power usage to the state of the grid. This is done by inferring the context from available data sources, namely data made public by the grid provider and weather data.
We construct a dataset by scraping publicly available data at 10-minute intervals for three months. We then model our assumptions about the ANM in terms of generation and demand, which we evaluate against our dataset. Inspired by the method of Exploratory Data Analysis (EDA), we investigate our assumptions and look for patterns in the data through a series of visualizations. From this we extract correlations allowing us to represent our initial model in terms of factors external to the Orkney power grid: Wind speeds, time-of-day and day-of-week. To address the curtailment situations not described by our models, we search for anomalies in our data set, both by visual exploration and by applying different anomaly detection rules. When redacting these anomalies, we see the ratio of time with any curtailment in the ANM reduce by over 50\%.
As a supplement to the manually constructed models, we also evaluate models based on Artificial Neural Networks (ANNs), finding a slight increase in model accuracy, but no increases in tangible understanding of the ANM, indicating that ANN-based models can be useful for deployment, but not for the EDA-process. We analyze the data collected by a single wind turbine and find that the majority (>90\%) of the loss reported does not seem to be caused by ANM-issued curtailment. Using the wind data generated by this turbine as input to our ANN-based model leads to additional accuracy gains. Finally, we combine our correlation-based model with publicly available weather forecasts to obtain a predictive model, allowing us to forecast future curtailment.
The models developed in this project can serve as indicators that a significant amount of the curtailment is caused by something other than the demand/generation balance, supporting the stakeholders feeling of an unclear logic governing the ANM. When supplying the models with the developed anomaly detection rules, they can be used to implement the power controller logic of an Orkney Cloud data center.